In a digital competitive landscape, it’s more important than ever to provide visitors with a personalized experience that caters to their unique preferences and needs.
This is why e-commerce websites often use special algorithms to display complementary or similar items to visitors to encourage them to make more purchases. You’ve most likely seen this before: „you might also like“ and „bundle these items“ are two algorithms that are very popular among e-commerce brands. It could also be in the form of emails sent to customers suggesting similar items to capture their interest and get them back on the website to complete another transaction.
They also provide opportunities for up- and cross-selling so as a user views one product these algorithms automatically offer suggestions for other similar items. Seeing items in the context of a complete set can entice customers to add more items to their cart, thereby boosting sales for the company.
This is the idea behind a product recommendation engine.
What is a product recommendation engine?
A product recommendation engine is a filtering system which aims to predict and display additional products to visitors that may be of interest to them.
A product recommendation engine tracks your visitors’ behavior on a website then, through a combination of machine learning and artificial intelligence (AI), it generates a list of product suggestions and other relevant offers or discounts most relevant to them according to their browsing and purchase history.
In other words, it’s a combination of information filtering and matching algorithms to find the right match between the user and content.
Therefore, the engine gathers large volumes of user data such as user behavior and to find patterns behind the behavior and actions of a user in order to generate valuable insights. These insights are key to displaying the most relevant content to each user.
The idea behind a product recommendation engine is nothing new. All the major brands from Netflix, LinkedIn to Amazon and Spotify implement this strategy to deliver suggestions based on data tracked and stored in their system.
With millions of products on sale on its website, Amazon relies on a number of recommendation strategies on its product pages to make it easier for customers to find what they need quickly and drive purchases as seen in the image below:
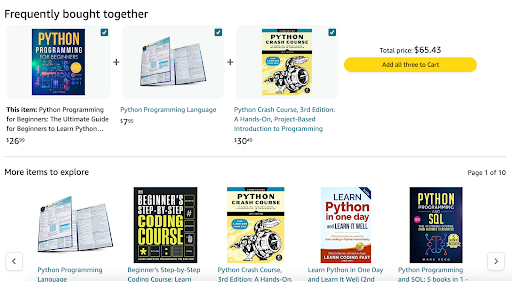
Based on what a customer is viewing and their browser history, Amazon will recommend similar items to encourage them to make another purchase as a “package deal” through cross- and up-selling to ultimately increase average order value.
Types of recommendation engine algorithms
Product recommendation engines typically rely on certain advanced algorithms drawn from customer data that include purchase history, preferences and search and browse behavior.
With that information, the recommendation engine will automatically generate a set of personalized recommendations for each customer based on this data. The system will continuously update as new information comes in regarding the customer to provide fresh, new recommendations.
Based on your business goals, you can utilize a variety of algorithms to influence website visitors with different suggestions. Let’s take a look at a few different algorithms that can be used based on the information collected by the engine.
Content-based filtering
In a content-based recommendation system, a user profile is built according to the items the user previously liked, purchased, searched for or clicked on. The keywords used during the search process are the foundation for the product suggestions.
Through machine learning, the recommendation engine establishes similarities between products and will then recommend similar products recorded in their history. This type of filter relies on the assumption that if the user liked a certain item in the past, they are more likely to have a similar reaction to a similar item.
Collaborative filtering
Collaborative filtering involves collecting large amounts of data from multiple users about their preferences and behavior and then predicting what an individual user will like based on similarity.
A big advantage with this type of filtering is that it doesn’t require information about the actual user or item per se; it only needs data about past user behavior.
In particular, as seen in the image above, Amazon relies on Item-Item collaborative filtering to boost sales. The Item-Item algorithm follows the logic “if you like this, you will also like….”. In other words, items are recommended to a user based on their previously-liked items by finding a “lookalike” item.
Meanwhile, the User-User collaborative filtering approach seeks to find “lookalike” users and suggest products to a user based on what their “lookalike” has chosen.
Hybrid recommendation system
As the name suggests, this type of recommendation system combines both content and collaborative filtering approaches. This system is highly effective as it brings together a complex mix of multiple algorithms while overcoming the downsides of each individual filtering model on its own.
Netflix is a great example of the implementation of the hybrid model as it seeks to base its recommendations both on how a user interacts with the platform (i.e their viewing history and highly-rated titles), content-based filtering, and what similar users have liked or watched in the past, collaborative filtering.
Transform your e-commerce website with an AI product recommendation engine
Eine Product Recommendation Engine ist ein hochwirksames Tool, um hochpersonalisierte Benutzererfahrungen zu liefern, indem Kunden relevante Inhalte gezeigt werden, die auf ihre individuellen Bedürfnisse und Interessen zugeschnitten sind.
Wenn ein solches System richtig eingerichtet ist, kann es einen großen Schub für Conversions und Transaktionen sowie andere wichtige Metriken bieten. Ein Empfehlungssystem kann auch einen großen positiven Einfluss auf die Benutzererfahrung haben, da jedem Besucher ein einzigartiges Set von Artikeln basierend auf seiner Historie gezeigt wird.
Mit anderen Worten, die Produktempfehlungen eines Besuchers werden sich von der Liste der Empfehlungen eines anderen Besuchers unterscheiden. So kann ein solches System die Kundenzufriedenheit und -bindung erheblich steigern. Empfehlungssysteme sind eine großartige Möglichkeit, dein Geschäft zu fördern und das E-Commerce-Erlebnis mit modernsten KI-Produktempfehlungen zu steigern.
Möchtest du eine Product Recommendation Engine zu deinem Toolstack hinzufügen? AB Tasty ist die komplette Plattform für KI-gesteuerte Empfehlungen, Experimente und Personalisierung. Mit eingebetteter KI und Automatisierung kann dir diese Plattform helfen, ein reichhaltigeres digitales Erlebnis zu erzielen.
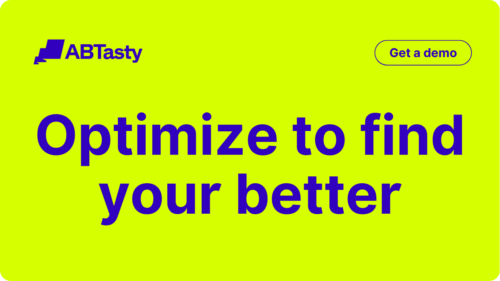
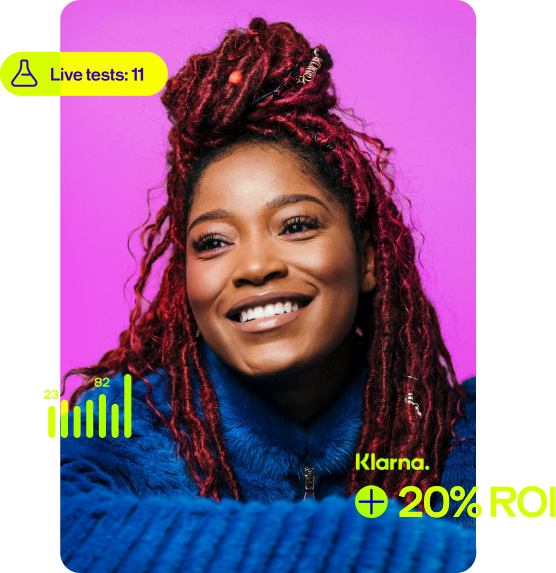
mit ABTasty
Erhalten Sie eine individuelle Komplettlösung für die Plattform
Demo anfordern